Energy is a significant cost in 5G telecom systems, and saving energy is good for the environment and business. In a typical 5G installation, 80% of the energy is consumed by the radio access network (RAN) equipment; the remaining 20% is needed for transport, core network functions like mobility management, routing, security, policy control, charging, and subscriber data management, and operation support system (OSS) functions. That makes 5G energy management as complex as it is important.
The International Telecommunication Union has established a Focus Group on Environmental Efficiency for Artificial Intelligence and other Emerging Technologies and issued a draft report, ITU-T FG-AI4EE D.WG2-02: Computer processing, data management and energy perspective. This FAQ provides an overview of the energy savings in 5G networks that can be enabled by artificial intelligence (AI) and machine learning (ML), looks at specific uses for AI energy savings in base stations, and closes by looking at energy savings enabled by AI in RANs.
AI-based energy-saving algorithms can be implemented with no impact on network performance and no large-scale equipment deployments or network hardware changes. A large amount of equipment in a typical base station runs in idle mode for much of the time. That makes AI-enabled dynamic shutdowns one of several important energy savings tools. According to Nokia, benefits of AI energy management for mobile networks can include:
- Up to 30% overall energy savings
- Up to 5 times more power savings than non-AI systems that perform temporary shutdowns based on fixed schedules
- Up to 70% less energy consumption for cooling.=
- Minimization of energy waste across active radio and auxiliary equipment
Dynamic shutdown is one of the most significant energy savings opportunities for AI in 5G. AI can handle complex cross-domain optimization problems and develop dynamic strategies. Networks generally have cyclical use patterns, for example, peaking during the workday and shrinking dramatically in the middle of the night, and using a fixed equipment shutdown can save energy. But those patterns aren’t absolute. Using AI enables network operators to develop predictive analysis based on parameter optimization at the application level, combined with ML training and optimization for model building and data cleaning and aggregation at the data processing level, leading to optimized data acquisition and proactive and improved configuration management instead of shutdowns on a fixed schedule (Figure 1).
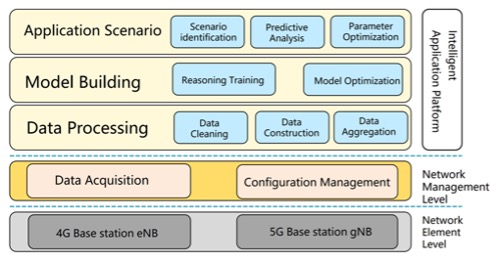
AI and RANs
RANs experience wide swings in traffic based on where they are located and the time. Efficiently managing the operation of various cells and shutting down unnecessary capacity is critical to minimizing RAN energy consumption. Not every cell in a RAN experiences the same loading pattern, and they need individualized energy management in real time. That’s where AI and ML come in to enable dynamic configuration changes across the cells to maintain a high quality of service (QoS) while reducing energy consumption by up to 12%. One possible approach to using AI and ML is shown in Figure 2:
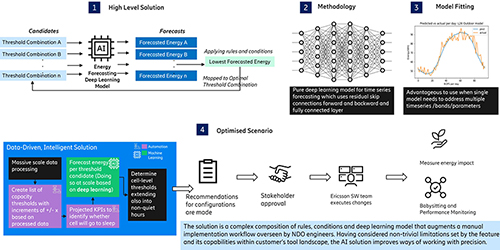
- The high-level solution consists of an energy consumption forecasting model.
- The methodology is the tool used to develop the optimal configuration threshold grid search model.
- Model Fitting identifies the preferred threshold validation on the network.
- Optimized Scenario performs an impact analysis to adjust the model as needed to support the required QoS.
Two key models in this approach are the forecasting and optimization models. The forecasting model works a day ahead to predict energy consumption for each cell. It provides the baseline for the optimization model, which identifies potential improvement in terms of performance criteria like accessibility, throughput, anticipated traffic volume, mobility, latency, and so on. AI and ML are used here to implement and refine a convex optimization model to maximize sleep hours subject to business and technical/performance constraints.
Summary
AI and ML provide powerful tools for energy management in 5G systems. The ITU has active programs to identify and develop AI-based tools to develop holistic approaches to data processing, data management, and energy management. Those tools can be used to develop energy-saving algorithms for individual cells and RANs.
References
Application of AI technology in 5G base station to Improve Energy efficiency, China Telecom
Focus Group on Environmental Efficiency for Artificial Intelligence and other Emerging Technologies (FG-AI4EE), International Telecommunication Union
How Artificial Intelligence reduces the carbon footprint of telco networks, Nokia
ITU-T FG-AI4EE D.WG2-02: Computer processing, data management and energy perspective, International Telecommunication Union
Optimizing energy efficiency with AI-powered energy management software, Nokia
Why AI-powered RAN is an energy efficiency breakthrough, Ericsson
Leave a Reply